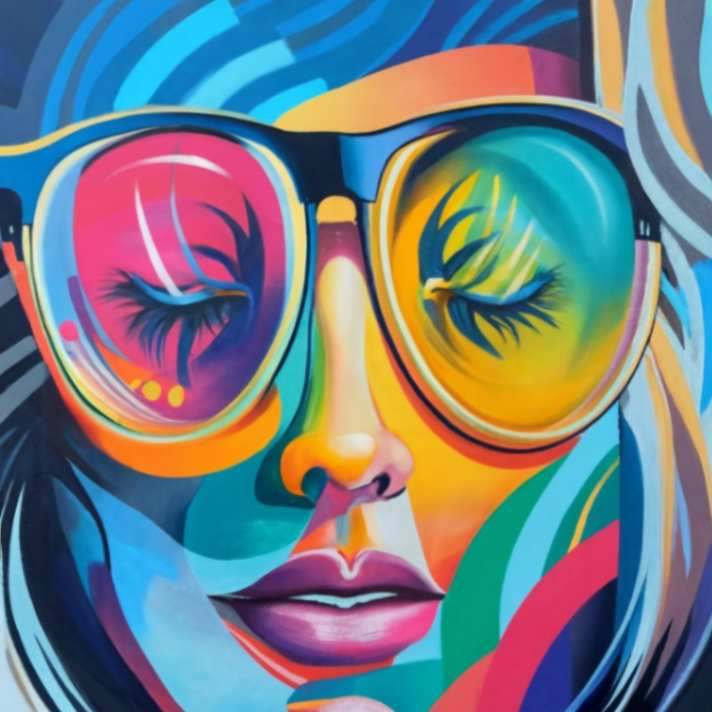
Title: A Comparative Analysis of Sleep Cycle Apps on Apple Watch and Scientific EEG Devices
In this article, we delve into the realm of sleep tracking and compare the efficacy of the Sleep Cycle app on Apple Watch with a small sleep EEG device used in various research projects. The aim is to directly compare the results obtained from both devices over six nights of testing.
The Sleep Cycle app, initially developed as a smartphone application, detects sleep stages by measuring movement. However, since May 2020, the app has also been made available for Apple Watch. The exact sensors utilized by the app remain unknown; the only visible data is the morning heart rate, leaving room for speculation on whether heart rate or heart rate variability are actively incorporated into the algorithm.
The Sleep Cycle app tracks the depth of sleep rather than the traditional sleep stages, showcasing a graph ranging from awake to deep sleep. Its primary objectives, as stated on their website, are to gain a better understanding of sleep patterns and to wake users up in the light sleep phase to facilitate a refreshed awakening.
To compare the results, the sleep stages tracked by the Sleep Cycle app on Apple Watch were juxtaposed with those recorded using a scientific EEG device called the Hypnoline Z-Max. Additionally, an infrared camera was used to observe movement patterns throughout the night. The EEG device measures brain waves and muscle movements and is commonly employed in scientific studies.
Analyzing the data from the Sleep Cycle app proved to be a slightly complicated endeavour as exporting the complete graph in a usable format proved impossible. However, with the assistance of an online tool called web plot digitizer, raw data could be extracted from screenshots of the graph.
The six nights' recordings were independently assessed and scored manually for different sleep stages using the EEG device. Each individual night was then compared to the Sleep Cycle app's graph.
In the comparisons, it was observed that the Sleep Cycle app's scores generally showed lower values during moments of deep sleep, aligning with expectations. However, discrepancies emerged later in the night where there was no deep sleep, yet the app still displayed lower scores. Similarly, during REM sleep, the app occasionally assigned higher scores, yet lower scores were observed when REM sleep was absent.
The infrared recordings provided an opportunity to validate the accuracy of the Sleep Cycle app in predicting wakeful moments during the night. By examining movements visually, it could be determined if the app correctly identified the instances of wakefulness.
Ultimately, this comparative analysis highlights both the potential of sleep cycle applications on wearable devices and the limitations they may exhibit. While the Sleep Cycle app on Apple Watch shows promise in tracking sleep depth and facilitating a peaceful awakening, it must continue to improve its precision in accurately identifying different sleep stages.